Using microdata in criminal justice
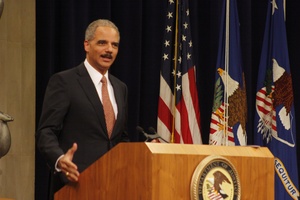
While the collection and release of individual-level microdata is hotly debated across social disciplines, microdata has greatly improved outcomes and decision-making in criminal justice programs.
In April, former Attorney General Eric Holder cautioned the implementation of microdata-based programs in criminal justice, saying that they could negatively affect minority groups. Indeed, privacy advocates have noted that the use of individual-level data can sometimes propagate patterns of discriminatory bias. However, Holder was fully supportive of the use of aggregate-level data. This opinion is widely reflected throughout the criminal justice system, including within risk assessment programs. These programs have generally found that the use of microdata can help mediate the risk of failure to appear at trial, recidivism and early prison release.
One of the clearest examples of the use of microdata mediating risk in criminal justice are in pretrial risk assessment programs. A study by the Laura and John Arnold Foundation established a public safety assessment (called PSA-Court) based upon nine factors that predict risk of recidivism or failure to appear at trial. These factors relate to the present case and a defendant’s criminal history, and not demographic data that could propagate discriminatory bias. Further, the factors did not require an interview with the defendant, allowing this risk assessment model to effectively reduce adjudication and incarceration costs, all while avoiding the subjective judicial process that often misplaces both low- and high-risk individuals in the system.
While microdata is used internally as a means of constructing these risk assessment tools, it can have damaging effects when made public. States like Nebraska and Texas make personally identifiable correctional data available online, amounting to an open record of every inmate in the system, their personal information, and their offense and detention details. Unfortunately, this level of transparency is much more likely to have a lopsidedly negative effect on minorities coming back from prison into society.
Therefore, an important component of risk assessment algorithms is ensuring their proper implementation, much of which involves mitigating any discriminatory predilection. To avoid the issue of minority bias in how these tools model outcomes, a review of how fairly they process individuals through the system is vital. For example, in 2012, the Montana Board of Crime Control funded an assessment of their pre-adjudicatory detention risk instrument that analyzed the tool through two main dimensions, one of which “pertains to racial and cultural sensitivity in assessing offender risk.” The review allows practitioners to maintain a stance on keeping juveniles, when possible, out of detention and in alternative programs without endangering public safety or unfairly targeting minorities.
“The momentum behind this trend toward the increased use of risk assessment instruments emerged out of criticisms of subjective and arbitrary decisions regarding the processing of youth in the juvenile justice system.”
The Oregon Youth Authority exemplifies this trend in juvenile justice. The authority established a risk assessment model for juvenile justice recidivism in 2011 requiring the collection of individual-level data for Oregon youth on probation or under community supervision. Alike to pretrial risk assessment studies, this research centered around identifying risk factors for juvenile recidivism to make cost-efficient supervision and treatment decisions.
In Hawaii, the collection and study of microdata was necessary to gain an understanding of parole program success. The Hawaii Parole Authority gathered individual-level microdata on over 300 offenders released on parole and tracked them for two years after their release. This study identified risk factors for parole failure that will inform smarter and cost-effective parole decision-making in the future.
Properly targeted programs have also helped reduce costs without compromising public safety outcomes. In fact, decades of research have shown that without data-driven assessments of offenders, the possibility of, for example, over-supervising low-risk offenders is likely to produce worse outcomes than essentially leaving them alone. Travis County, Texas, considered a pioneer in the use of such strategies, was pushed toward evidence-based practices after the legislature convened in 2007 facing a major dilemma. As a rapidly growing prison population brought state facility capacity to its limit, lawmakers considered spending a half-billion dollars to build more beds that would accommodate the surge. Instead, a bipartisan coalition of leaders opted to explore diversion programs aimed at reducing recidivism through models based on data. Developing these models involved properly identifying the population and matching supervision strategies to the population profile. While such a fundamental change in procedure was not simple and required methodically revamping probation in the county, the results were clear: Revocations, absconders and rearrests all slowed following the model’s implementation, avoiding massive fixed costs in prison construction.
“The diagnosis uses scientifically tested tools as opposed to the prior open-ended narrative of a presentence investigation report that could be written and interpreted in many different ways.”
Recidivism studies also pose a unique problem for individually identifiable correctional data. These studies are incredibly important as measures of success for time served, although some may argue that the department of corrections should not be allowed to keep data on inmates after their release. However, without long-term and individual-level monitoring of offenders, recidivism data would not exist. A balance is found in the aggregation of longitudinal microdata that helps to reveal recidivism trends without compromising privacy. An example of this is the Washington Department of Corrections’ Recidivism Rate Outcomes report (Note: Clicking on this link will download the report) that provides aggregate statistics on inmates released three years prior (the average length for recidivism studies). The Indiana Department of Corrections releases aggregates of even more sensitive microdata in its annual Juvenile Recidivism report. Despite the sensitivity surrounding juvenile data, the efficacy of juvenile correctional programs — and associated juvenile recidivism rates — are necessary to understand.
While individual-level data can allow for a longitudinal study of individuals, some data is best kept aggregated for privacy reasons. The New York State Office of Mental Health reported aggregate statistics from 2007 through 2012 on mental health inmate-patients housed in solitary confinement. Due to the health-privacy concerns, the data was aggregated. In this case, aggregation is the best method to release this data, as individual-level data would inappropriately disclose personally identifiable information as well as confidential health records. Aggregation is also a better method than nondisclosure of data on inmate-patients in solitary confinement. This report is an excellent example of how to release public-interest data while maintaining privacy in order to assess how correctional departments operate.