Avoiding prejudice in data-based decisions
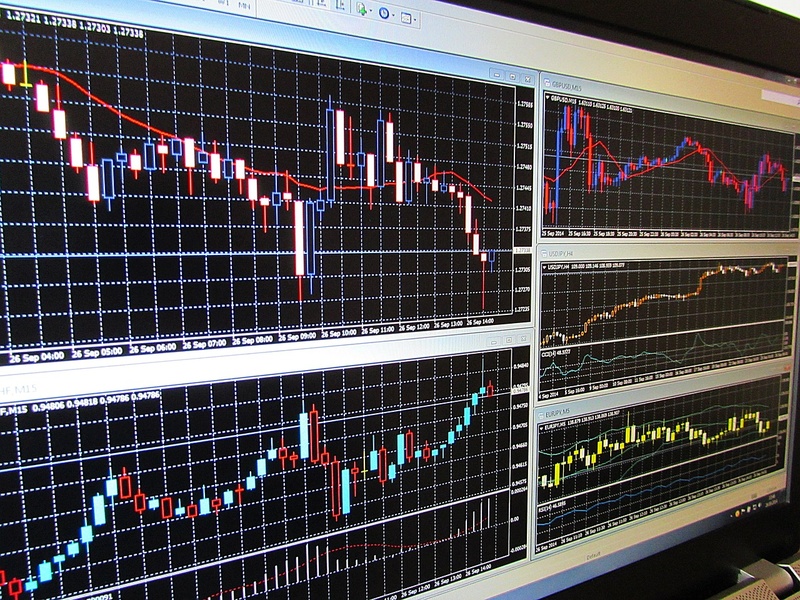
It’s important to know the potential problems of releasing individual-level data that can arise from bad data management practices, as well as the practices we can implement to lessen the likelihood of individual harm. At the same time, we should also think about the problems of the release of microdata — individual-level data — in broader context. Beyond the problems faced by specific people whose private information is incorrectly released, advocates have observed regular ways that microdata release can heighten risk of harm for specific communities. A series of projects issued over the last several years have provided essential texts for thinking about how microdata — as collected into “big data” — increases existing patterns of societal discrimination.
The essence of these arguments lies in an important, and perhaps counterintuitive observation: Using data and technology in a decision-making process doesn’t make a decision automatically free of problematic (and possibly illegal) social discrimination. Advocates have observed that in a number of situations, the additional collection and use of individual-level data can result in an entrenchment of discriminatory patterns, even as it becomes harder to see how this is done. The “big data” that’s used for algorithmic judgments about financial risk, housing, insurance or employment fitness invisibly incorporates the effects of human prejudices. As a result, relying on these large datasets to operate without oversight can lead decision-makers to discriminate against people who are already more likely to face discrimination, even while these data-based judgements stem less obviously from human prejudice.
In order to prevent data-driven decision-making from reincorporating patterns of prejudice, it is essential that datasets and algorithms be evaluated and audited for potentially discriminatory effects. Reviewers should consider how data collection, machine-learning processes and training materials, and category definitions might introduce — even inadvertently — elements of bias to the analysis.
Because data stemming from criminal-justice-related events are often legally used to prevent people from accessing social goods — such as the right to vote, certain kinds of employment, or places to live — the question of whether released individual-level data can systemically harm traditional subjects of discrimination looms particularly large. Arrest data provides a good example: Because individual-level, identified arrest data can create additional problems for people who are identified within the dataset — impacting their ability to get employment, housing or credit — the release of this data may especially disadvantage people from groups that are disproportionately arrested. Particularly because arrests are not the same thing as convictions, the release of this data can incorrectly label an individual as criminal.
Just as potentially problematic as data collection and release is the nontransparent use of microdata in automatic and nontransparent decision-making. Automatic decisions, produced by computer algorithms, can effectively be discriminatory when those algorithms were developed on the basis of discriminatory materials. Algorithms are built through the use of “training sets” drawn from past, human-made decisions. Algorithms to determine who constitutes a good employment candidate, for example, are drawn from lists of characteristics of existing employees. If humans regularly made discriminatory employment choices in the materials used to develop the algorithm, the computer will as well.
The potential for microdata use and collection to regularly, if inadvertently, amplify the effects of social discrimination is a serious problem associated with microdata release, and several recent projects on rights problems in big data have articulated ways for data managers to approach the problem. One valuable collection of materials is available in concert with a year-long set of projects launched through the UC Berkeley Center of Law. In April, a symposium entitled “Open Data: Addressing Privacy, Security, and Civil Rights Challenges” brought together legal, technical and privacy scholars to consider the specific problems that open data posed in connection with privacy law and principles. Another valuable collection of perspectives was developed by the New America Foundation’s Open Technology Institute, which articulated a range of specific concerns in Data and Discrimination: Collected Essays. Although a separate legal regime, the comparative perspective offered by Keiron O’Hara’s examination of the problem in British context can nonetheless be useful.
Perhaps the most comprehensive set of recommendations in this area have been put forward by the Leadership Conference on Civil and Human Rights (LCCHR). Organizing a group of leading civil rights organizations, the LCCHR identified a variety of concerns about how big data (including big criminal justice data) negatively affects communities of color. The Civil Rights Principles of the Era of Big Data release puts forth a set of new rights to protect in order to prevent the following systemic problems.
Profiling
Just as the suspicion of criminal activity based on race is called “racial profiling,” advocates term the uncontrolled development of suspicion of people based on their noncriminal data patterns “high-tech profiling.” In the law enforcement context, this problem plays out in close connection with “predictive policing” programs: As a result of having greater access to social media and other noncriminal datasets, police departments are able to compile lists of people who they suspect of being likely to commit crime — even if they have not yet done so. Last year, for example, The Verge reported on how the Chicago police alerted individuals that they were being watched because of their high propensity for criminal activity, even if they had not committed any crime, purely on the basis of the data collected about them. Without good protections in place, this sort of informative targeting of individuals could devolve into real harms to identified “pre-crime” suspects. Compare this example where the profiling that is occurring is not connected with the threat of immediate penalty, but with additional support and services: Richmond, Calif., is improving its homicide rate through targeting identified high-risk community members for additional support and services, while keeping this program separate from regular law enforcement.
Discriminatory automatic decisions
Taking the problem of problematic data-based decision-making one step further, human decision-making is sometimes removed altogether from important administrative processes and replaced with an algorithm. Particularly when this problem is combined with stigmatized social categories — like people identified as “convicted felons” — correction can be challenging and the impact can be consequential. The purging of over 50,000 voters from Florida’s list of eligible voters in advance of the 2000 elections offers an important example of how unsupervised algorithmic decision-making can exacerbate existing social discrimination. When given the instruction to develop a broad, “fuzzy-matching” list of potential felons from Florida’s voter list, ChoicePoint/DBT Online produced a large dataset; but Florida officials did not independently verify its accuracy, and tens of thousands of voters were disenfranchised. Many of these people were from already politically underrepresented groups.
To prevent discriminatory judgments of particular community groups, advocates recommend the use of independent algorithm audits to discover whether decision-assisting computer patterns are providing novel insights — or are mainly reinforcing discriminatory patterns. According to “An Algorithm Audit,” the authors write, “Testing by an impartial expert third party … [can] ascertain whether algorithms result in harmful discrimination by class, race, gender, geography, or other important attributes.”
Lack of control over personal information and inability to correct inaccuracies
Since the birth of a major movement to improve privacy law in the 1970s, a core principle of government data use has been the requirement to provide “notice and consent” — that is, to provide information to people so they know the use to which their information will be put, and to give them the option to refuse. The full list of “Fair Information Practice Principles” (FIPPs) includes a number of specific ways that individuals can be guaranteed fair notice and access to their information. Implementation of these practices remains the best goal for any data collection or aggregation effort, but these guidelines are very frequently disregarded both by public and by private data managers. Indeed, the problem of precisely how to best implement notice and consent principles in the current technological environment remains pressing and unsolved.
Where data are being collected and used without transparency, inaccurate or illegal uses of data also become invisible. Current documented illegal uses include cases that result in criminal charges, including LeapLab’s sale of collected personal financial data from hundreds of thousands of people to an entity that withdrew money from people’s bank accounts. Inaccurate data can be particularly harmful to individuals. For example, legal cases have arisen from data brokers sharing data that included the false status of individuals as sex offenders.
Advocates are especially concerned about how nontransparent means of data collection by both governments and private actors ultimately produce storehouses of large, complex datasets about individuals, which then get used in new and potentially discriminatory ways. Commercial data brokers, who collect, package and resell access to personally identifying data, have been the subject of official concern for a long time. Indeed, the Federal Trade Commission (FTC) identified “the lack of transparency among companies providing consumer data for credit and other eligibility determinations [as leading] … to the adoption of the Fair Credit Reporting Act.” In 2014, the FTC issued a new analysis of the current risks and benefits to American consumers posed by data brokers who use their billions of pieces of personal data to guide marketing, assess risks and detect fraud. They observed that, currently, “many data broker practices fall outside of any specific laws that require the industry to be transparent, provide consumers with access to data, or take steps to ensure that the data that they maintain is accurate.” The FTC’s recommendations to mitigate these risks include passing legislation to require data brokers to adhere to notice and consent principles about their data holdings, including with new approaches to how this works for large data aggregations.
For the law enforcement community, these recommendations are particularly important in connection with data brokers’ risk mitigation and people-search services, since the problems of nontransparent practices by data brokers affect not only consumers, but also government agencies. Law enforcement agencies contract with data brokers to obtain information about the communities they police. This practice — contentious for many rights advocates, who see it as potentially evading legal warrant requirements — was most publicly exposed in connection with the FBI’s contracting with ChoicePoint to access their data about all American consumers beginning in 2002. (ChoicePoint was acquired by LexisNexis and currently operates as LexisNexis Risk Solutions, marketing itself directly to law enforcement.) The problems of inaccurate data become greatly magnified when these data are used in law enforcement activities.
—–
As we increasingly come to depend on data and technology in our public and private decision-making, it is critical to know that while using a computer can produce important value, it does not guarantee us a substantively “objective” outcome. Because of the way that data are collected and interpreted, it is certainly possible for the additional use of individual-level data to reinforce existing problematic patterns, if users are not aware of the potential for this to occur.