How governments are safely opening up microdata
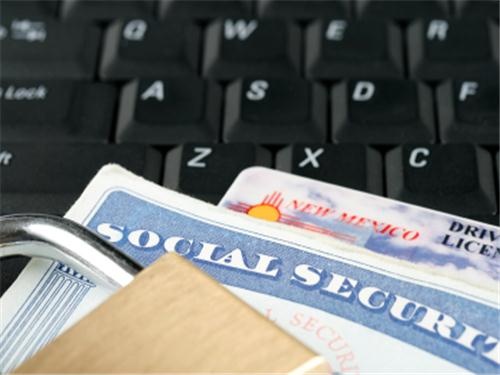
Over the past year, we’ve looked at different approaches to the question of how to open individual-level data — microdata — in the criminal justice context. While there are clear benefits to making individual-level data more available — such as better public analysis, improved data quality, and improving community relations through transparency — there are also significant obstacles. Despite these challenges, we see that governments are creating policies, processes and structures to help themselves accurately assess risks, address the challenges they find and publish new data openly.
These new policies, processes and structures offer important ways to address the risks related to the publication of microdata. Microdata is often valuable precisely because of its individual-level variation. Because individual rows of microdata are potentially unique to specific, identifiable, real-world individuals, this type of data release does risk increasing the amount of information readily available online about specific people. This is not a small challenge. Based on current practice, it appears that we cannot currently render microdata fully and irreversibly anonymous through solely technical means. We’ve considered alternate strategies for microdata use; for example, through formal partnering with external researchers who use identified data and then create analyses for public consumption.
Governments, meanwhile, recognize that they face both potential benefits and risks from microdata release. They must strike the right balance between being open and being protective. Increasing the availability of public data about private individuals can have potentially serious consequences, as was demonstrated in the case of how previously public motor vehicle registration information was used for stalking and murder. On the other hand, increasing the granularity of publicly available data can allow people to see the information that governments use for decision-making. It can increase the reach of the raw materials that researchers and analysts need to produce useful insights. It can spur concrete problem-solving that engages the public and creates a common ground for public discussion.
Identifying the right balance of public interest and protectiveness is particularly salient in the context of criminal justice data. This is an area of data for which there is strong and consistent public interest. As a result of legal precedent, grounded in our common law reliance on public trials, most information about individuals’ status in the criminal system is formally public. Public employees connected to the criminal justice system also are the subject of significant public interest, from police officers to judges to prosecutors. Much of these employees’ work, as well as aspects of their employment information, is also public, made accessible through public records and open meetings laws (although these laws also feature many exemptions, a number of which are uniquely enjoyed by law enforcement). Having access to this information can be valuable in a number of legitimate contexts, yet we also have legitimate worries about whether improving the accessibility of information will increase the potential for the individuals identified in these records to be harmed.
Given this concern, yet also being aware of the benefits of making microdata available for public use, governments seek to strike a balance that preserves as many benefits as possible while also preventing negative outcomes. As they seek to make public data more available for public use, governments are already figuring out regular ways to do this. As we discussed earlier, the publication decisions made in connection with California’s OpenJustice project demonstrate how one government agency found a balance between providing and withholding potentially sensitive details. Other governments are similarly identifying legally and politically possible points where they maximize data availability while minimizing harm.
In thinking about the strategies that governments have already implemented to balance openness and protectiveness — both within and beyond the domain of criminal justice data — we see certain themes regularly appear.
Establishing strong data governance
The first regular practice that governments employ in order to achieve a good balance of openness and privacy is to develop an effective data governance structure. A data governance structure that’s capable of setting data publication policy and practice ensures that data collection, review and publication can be managed in a way that improves both the quality of the product and confidence in the program.
Data governance truly touches every aspect of the question of balancing openness and privacy. A person or group must be charged with developing the jurisdiction-specific questions that the government wants data managers to answer before releasing datasets. Similarly, an individual or group of individuals must be charged with helping departments continually follow established data processes. Without making someone specifically responsible for making sure that data is ready for release — in terms of its quality, completeness and appropriate redaction through a regular and transparent process — a government may stray into ad hoc data release processes which are not sustainable and may result in errors, setting the whole project back.
Although a variety of internal managerial roles have been assigned to perform this function, there is an increasing trend to assign this set of responsibilities to a Chief Data Officer (CDO). Outside of government, the CDO role was developed to help companies ensure compliance with requirements for managing specific types of federally regulated data, including health care and consumer data. While the CDO is certainly responsible for establishing good data management practices, they are also increasingly developing and driving data strategy: that is, identifying how the organization’s use of data can help it better achieve its goals. When it comes to the question of whether to release data that is sensitive but of public interest, having a manager with an eye on overall data strategy helps ensure those decisions are well informed.
Current municipal CDOs have written publicly about their data management policies, and many agencies (or new CDOs) can draw on these resources as a way to begin thinking about implementing or communicating about data governance practices. Two good repositories for this information are:
- DataSF resources by Joy Bonaguro, San Francisco’s CDO
- DataLA’s Open Data Policy, particularly “How We Open Data.”
Another approach to setting good parameters for data release lies in creating an open data advisory board that’s charged with developing data management and review practices. Particularly in the early stages of an open data initiative, this is likely to be the easier way to ensure good management of the program, since it locates decision-making in existing governmental leadership (and also avoids the need to hire new staff). The Center for Government Excellence at Johns Hopkins has produced a useful resource for learning how to set up and run an open data governance committee.
Identifying legally restricted and sensitive data
A key task of a data governance process is to ensure that the government publishes no information which it is legally prohibited from publishing. In addition to federal privacy laws that apply to all U.S. states and localities, each state (and a number of cities) has its own set of laws describing legal prohibitions on the release of individuals’ personally identifying information (PII). To get an overview of conditions across all states, Robert Ellis Smith provides a useful and regularly updated compilation of laws related to the protection of personal information. Experts have also compiled 50-state overviews of law in specific data areas, such as this overview of health data privacy created by the Health Privacy Project, this review of state data breach laws (with definitions of restricted data) by BakerHostetler and this scan of state student privacy law by the Foundation for Excellence in Education (as well as this complementary study of recent student privacy bills by the National Conference of State Legislatures). To look at just laws in a single state, some, like California, Wisconsin and Massachusetts, provide all relevant data privacy laws on one page, while others require a search through state statutes.
There is a clear imperative to review local law in determining whether it is legal to publish each element of a dataset. Meanwhile, it is also useful to review data being considered for publication for its sensitivity. “Sensitive” data is data which it is legal to publish (e.g. would be returned through a public records request), yet which a thoughtful review reveals may potentially have some privacy implications for individuals. The DataSF Handbook identifies data as sensitive if “in its raw form, this data poses security concerns, could be misused to target individuals or poses other concerns.” The DataLA policy handbook uses a more expansive definition, identifying “sensitive data” as having one of the following qualities:
- Sharing the data has not been mandated by the legislature, an auditing entity or other entity outside the participating department.
- The data table has implicit or direct policy implications.
- The data table is likely to attract media attention (either positive or negative) or is subject to ongoing media interest.
- There is legislation pending or recently passed related to the data table. A legislator has held or scheduled hearings on the content area of the data table. The data table will likely attract legislative interest. There is pending enforcement action or litigation related to this data table.
These filters are important for knowing the potential impact of a publication decision. However, unlike legally restricted information — which a government should not publish — a government very well might want to publish sensitive information. There can be significant reasons in the public interest to release information which has been deemed to be sensitive; for example, if releasing the information helps shed light on government practices which are undergoing public debate. Moreover, since it is legal to publish public records, information which is not legally restricted can always be published by an outside party; sufficiently interested private individuals could make public records requests and publish the sensitive data on their own. In those cases, it could be better for the government to publish data first and be sure it was done well, adding contextual information as appropriate.
Once sensitive information has been identified, reviewers can perform a balancing test to determine the relative costs and benefits to publishing the information. Tests which balance the cost of releasing private information against the public’s right to know have developed over the history of U.S. Freedom of Information Act case law, and might be good as a starting point. They include the two-factor test and the more detailed five-factor test, which evaluates:
- Whether disclosure would result in a substantial invasion of privacy
- The extent or value of the public interest and the purpose or object of the individuals seeking disclosure
- Whether the information is available from other sources
- Whether the information was given with an expectation of confidentiality
- Whether it is possible to mould relief so as to limit the invasion of individual privacy.
These considerations could be worked into a review of sensitive data for an open data program as well.
A very interesting, if more restrictive, approach to sensitive information has been developed by the city of Seattle within their new city Privacy Program. Through their Privacy Program, Seattle works deliberately to become aware of and minimize the privacy implications of their data collection. The program does this though taking a rigorous and thoughtful approach to data governance, designing a data management structure that is capable of upholding their city-wide Privacy Principles. As city employees who collect personal data work through the city’s Privacy Review Process, they are asked to review their program’s potential for violating the Privacy Principles. They begin with a quick Self-Assessment to determine whether their program has implications for public’s privacy rights. If it does, and the department’s Privacy Champion can’t adjust the program to eliminate those implications, the manager conducts a much more comprehensive Privacy Impact Assessment, which is “designed to outline the anticipated privacy impacts from a City project/program or project/program update that collects, manages, retains or shares personal information from the public.” Using this approach, it seems unlikely that sensitive data will ever be published without a full and careful balancing of the competing interests at stake.
The data review process recommended in San Francisco, meanwhile, provides another structured approach to consider. In San Francisco’s case, each department’s data coordinator is required to maintain an inventory of the department’s internal data. Coordinators catalog a number of observations for each dataset inventoried, including a full list of data elements, a listing of any privacy laws which might govern those data elements and a consideration of any ways that those data elements or their combination might be viewed as sensitive. These observations are then used to determine whether the data will be published, and, if so, which data transformation techniques will be used to mitigate risks or comply with prohibitions.
Mitigating risks in specific datasets
An effective review process, established through a strong data governance structure, will make a government confident that it can identify data which is legally restricted or too sensitive to publish in raw form. Once this has been done, governments then apply techniques to remove the restricted or sensitive elements from the data they’d like to publish.
Some of the most common approaches to excluding nonpublishable information include:
- Aggregating raw data. While individual-level data provides significantly more utility for use in detailed analytic work or software applications, it is often preferable to have aggregations of data points than none at all. Consistent aggregation allows for the mapping of trends over time and comparison across geographic location. Aggregation is a common technique when looking at identifying information like address points or highly regulated information like health and mortality. This example from the Baltimore Neighborhood Indicators Alliance demonstrates how local data can be aggregated to provide an estimated rate that could be used by others without revealing any protected information; geographic information is often aggregated to the block or census block level. Since aggregated data appears frequently in reports, it is important that data holders also remember to provide downloads of the aggregated data alongside in open, structured formats alongside reports so that the data can be freely used.
- Redacting specific fields. Where concerns about releasing data center on the problem of having fields or columns which contain PII, it is possible to provide the remainder of the individual-level data without the problematic columns. Redacting fields that contain PII makes the nonprivate aspects of the data available for public use, while withholding the most obvious and unambiguous methods of identifying individuals (e.g. through their names, addresses, birthdates and social security numbers). Seattle’s example categories of personal information provide a slightly wider variety of fields to consider for redaction.
- Suppressing low-frequency data. After specific and highly identifying fields are removed from data, it can still be possible to identify individuals if there is only a small number of individual cases which share specific characteristics (“small cells”). As a result, public datasets can be made more protective of privacy if individual, unusual cases which demonstrate fairly unique patterns are suppressed. For example, if only a few rows refer to a need for a specific disability accommodation, it’s possible that someone familiar with the population being studied would know who was being referenced in that data. For that reason, data publishing guidelines will sometimes say that any characteristic or field that contains fewer than five cases should not be published, or that additional review should take place when there are fewer than 10 cases.
- Improving collection practices. While we think quite a bit about the publication side when it comes to balancing protectiveness and openness, it is also important to think about reducing the amount of PII that government have to manage in their data. This can be accomplished by focusing on improving data collection practices and limiting the amount of PII that is collected in the first place. Preventing the collection of unnecessary PII is a strategy that Seattle has prioritized as an aspect of its Privacy Program; its review process actively encourages program managers to consider what private data they could avoid collecting through their work.
Another aspect of improving data collection for preventing incidental PII collection is to prevent the inclusion of “accidental” PII — that is, PII that the government did not request, but which was submitted anyway. The U.S. Census Federal Audit Clearinghouse, which collects Comprehensive Annual Financial Reports from state and local governments as well as nonprofits, had experienced submitters inappropriately including Social Security numbers in their public submissions. To address this, they changed their submission form to require that the submitting auditor testify that they had included only the required data elements and information.
A final way that collection can be improved is by notifying the people whose data is collected about the management and use of their data. Seattle’s practice here leads again: Through its “Full Privacy Statement,” made available on its website, Seattle offers an excellent example of how a city can respect residents’ rights to “notice and consent” in connection with the city’s data collection and use.
In sum, although there are challenges to opening data that contains sensitive or private elements, there are a number of regular strategies that governments employ in order to find the appropriate balance between openness and protectiveness. Finding this appropriate balance is likely to be an ongoing process, one that changes over the life of the open data program. However, with the establishment of good data governance, the implementation of processes which aim to identify potential problems with publication and the use of thoughtful risk mitigation techniques, governments can have confidence that they are addressing those challenges head on.